Fostered by advances in IoT technology, Sailbri Cooper’s SCI-608 air quality sensor network monitor uses machine learning algorithms, which are fully deployable on a cloud platform, to conduct in-situ calibration of the data. Our algorithms and calibration systems have been proven to be reliable during high pollution episodes. The periodic wildfire episodes in the West Coast during recent years have been threatening the ambient air quality complement to traditional spatially dispersed air-quality networks, which measure high spatiotemporal variations of air pollutants. In situ air pollution monitoring, data can inform the public about the ambient air pollutant concentrations in a timely manner when a wildfire occurs, so that people or local government can quickly react with safety measures to reduce individual personal exposure.
During a wildfire episode, the PM and CO concentrations can reach more than 100 times of normal concentrations. Such high-pollution concentrations impose additional challenges in the low-cost monitoring field, especially when the low-cost sensors are typically calibrated at normal concentration levels. The SCI-608 air quality sensor network monitor uses machine learning algorithms, which are fully deployable on a cloud platform, to conduct in-situ calibration of the data. The sensor node’s algorithms and calibration systems have been proven to be reliable during high pollution episodes. Since August 2nd, 2018, two SCI-608 have been co-located alongside a research reference level nephelometer (Radiance Research M903, light scattering principle) at the SE Lafayette in Portland, Oregon (AQS ID: 41-051-0080). After the first and public health. Our sensor product is an important week of installation, the two sensors experienced periodic episodes of poor air quality due to wildfires indicated by significant increased PM2.5 and CO concentrations. The SCI-608’s machine learning algorithm uses historical data to optimize the prediction model and calibrates the data. Figure 1. demonstrates the hourly averaged nephelometer and SCI-608 PM2.5 concentration readings during the wildfire season of 2018, in which two wildfire episodes were observed amid 08/14/2018 and 08/22/2018. The two SCI-608 sensors did not accurately capture the high PM2.5 concentrations during the first wildfire event (over 20% difference between the sensors and the reference method). The difference between the reference measurement and the sensors triggered an automatic in situ calibration of the SCI-608. The linearities after each in-situ calibration are shown by the subplots on the right side of Figure 1. The improvement after each calibration can be clearly observed (as indicated by the increasing R squared). After being calibrated with the data from the first wildfire case, two SCI-608 sensors’ performance was significantly improved in the second wildfire event.
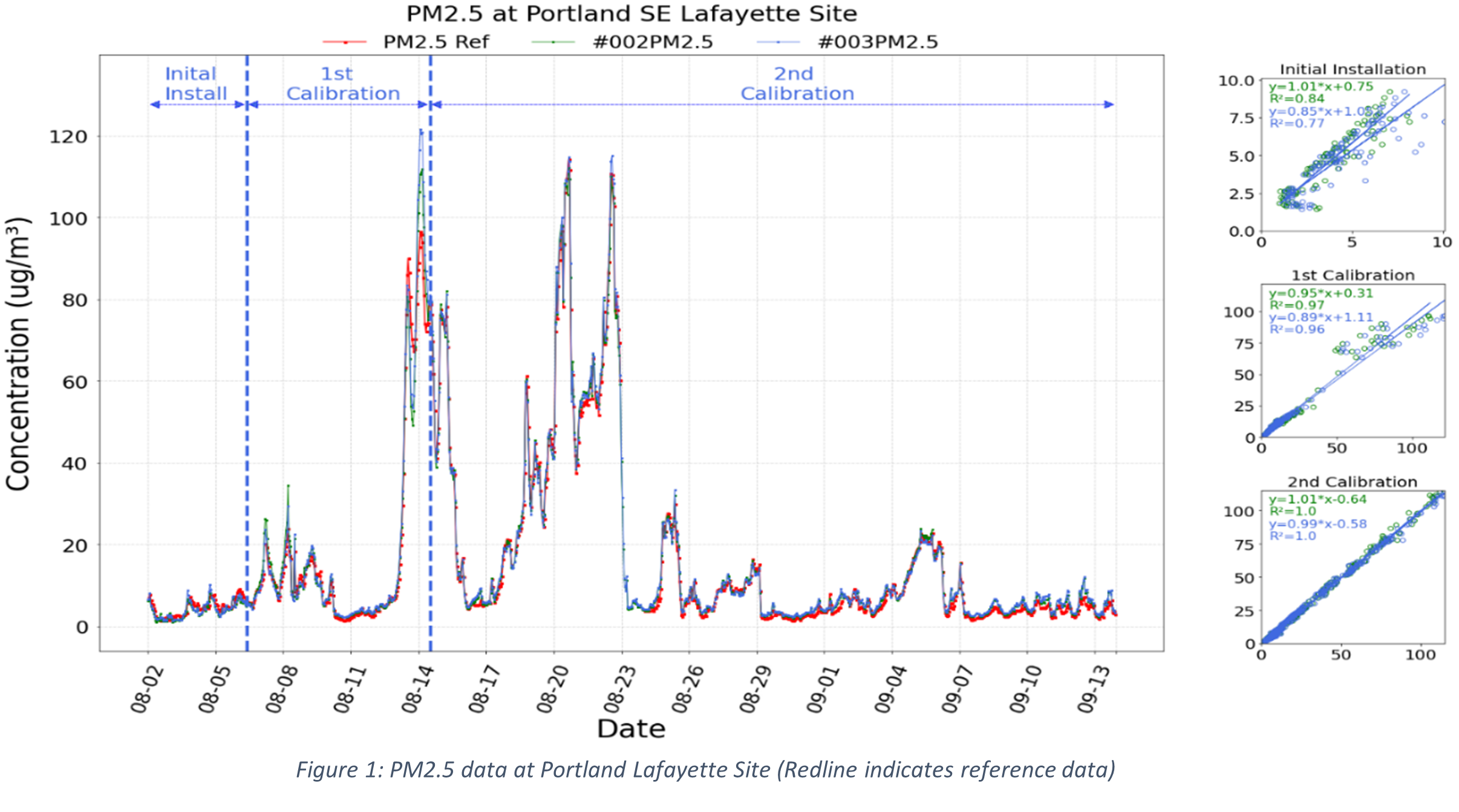
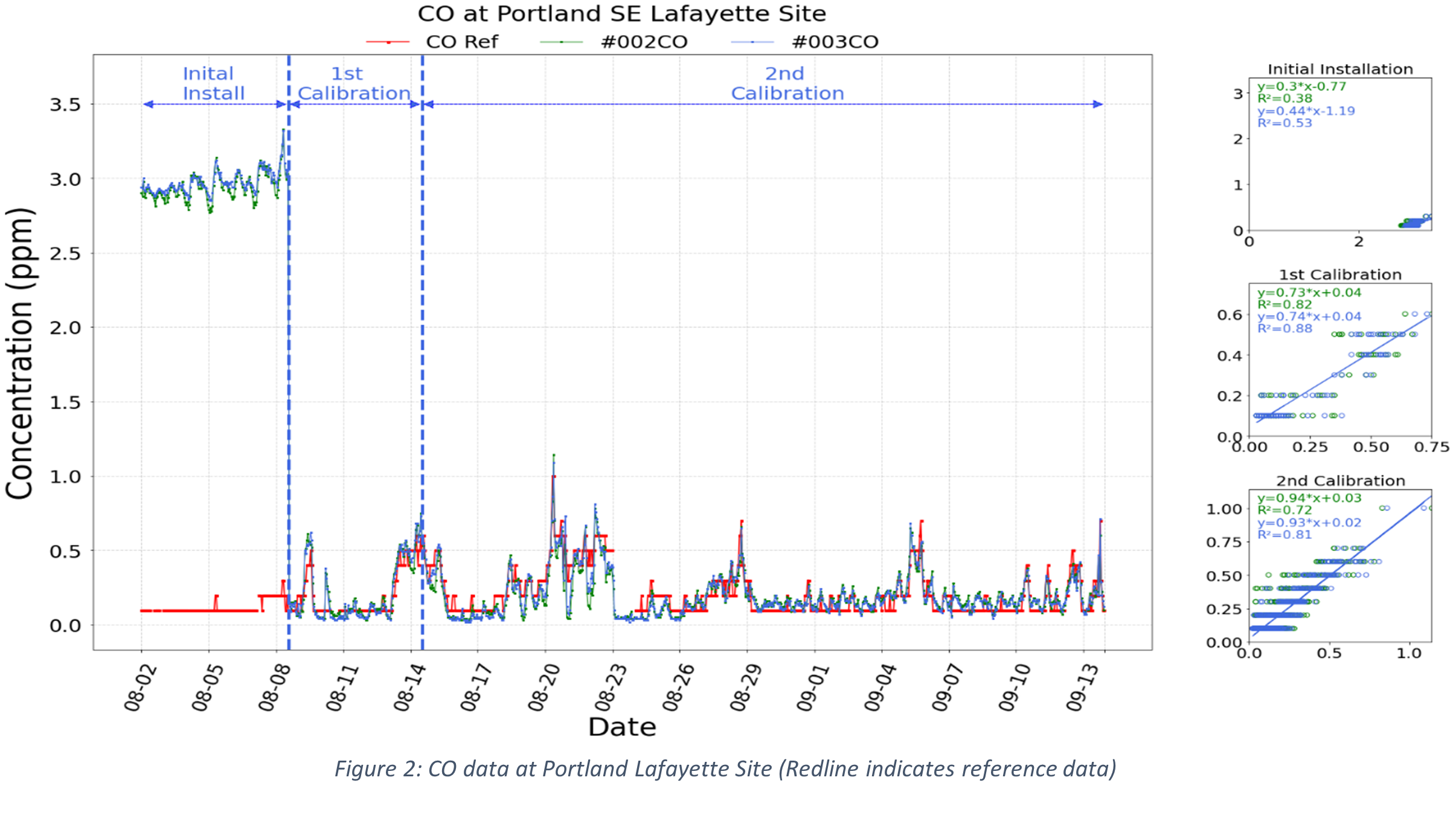
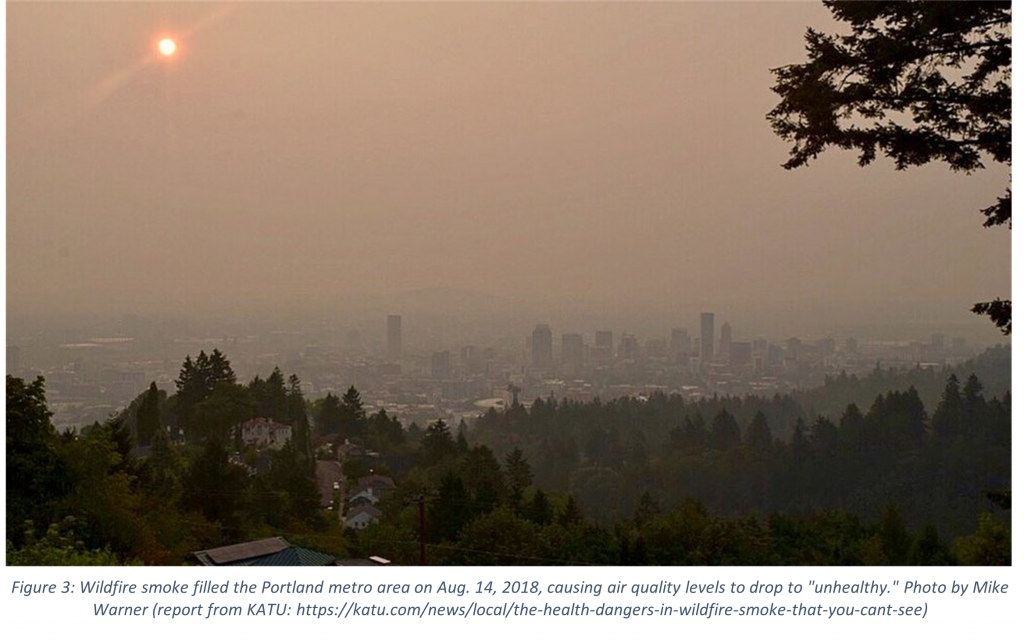
Long-term data evaluation is a typical method to assess the performance of air quality sensor products. It has been proven that sensor measurements can be influenced by seasons and various meteorological conditions. These influences are common causes of poor data quality. Using long-term evaluation data, one can observe the stability of sensor performance and determine the extent of seasonal and meteorological impacts. SCI-608sensor technology minimizes the impact of seasonal and environmental changes to provide robust air quality data.
SCI-608 air quality sensors have been deployed in multiple locations for evaluation purposes. For the two SCI-608 sensors at the Portland SE Lafayette site, a long-term evaluation had been performed throughout the whole 2019 (from January 1, 2019 to January 1, 2020). As shown in figure 4, both sensors demonstrated excellent correlations with the FEM for O3, CO, and particular matter (PM2.5), which indicates that the SCI-608 is robust and consistent. We also deployed two SCI-608 units at Queens College in New York City for three months around the end of 2019. The sensor data was compared to FEM measurements of PM2.5, O3, NO2, and CO. As shown in Figure 2, and the averaged R squared of two units with respect to reference instruments was 0.89 for PM2.5, 0.90 for O3, 0.95 for CO, and 0.83 for NO2. The results indicated excellent consistency between SCI-608 and FEM methods.
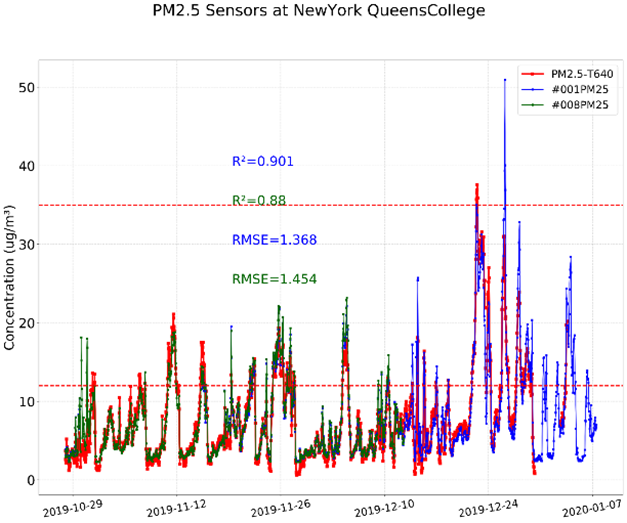
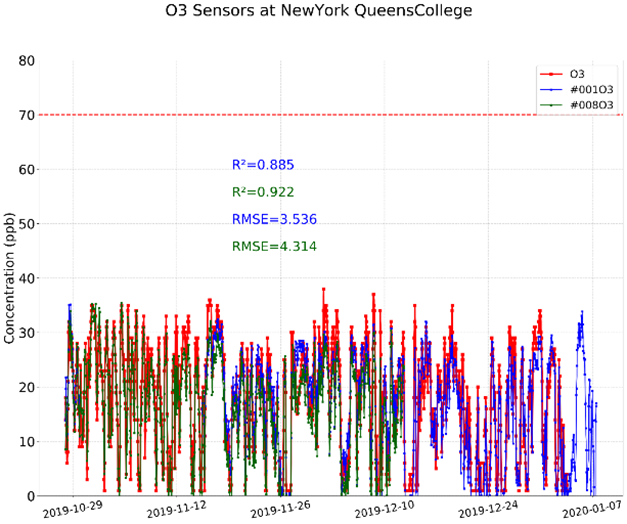
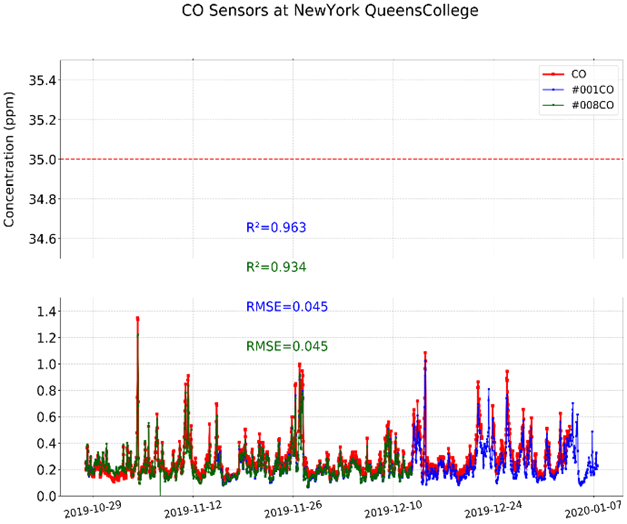
Figure 4: SCI-608 data in the city of New York (Queens College Site)
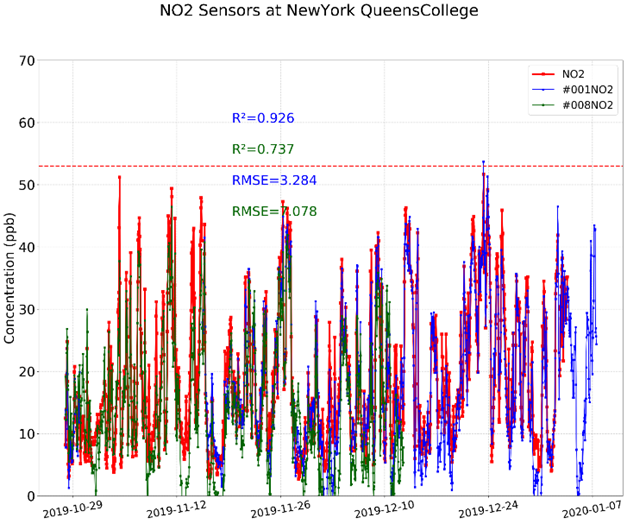
If you want to learn more about our sensor technology, please contact Dr. Yi Li at yili@sci-monitoring-cn.com.
Comments are closed